Quantitative trading in Singapore: An advanced guide to algorithmic strategies
4 min read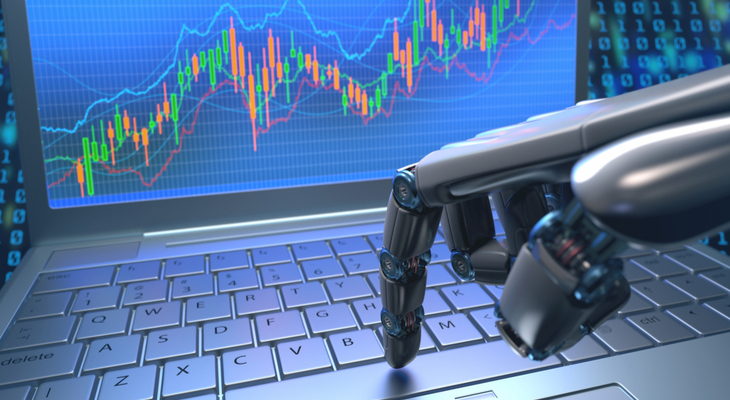
Quantitative trading, powered by algorithmic strategies, has revolutionised how financial markets operate. In Singapore, a hub for financial innovation, applying quantitative methods has become increasingly prevalent.
This article aims to provide an advanced guide to quantitative trading in the Singaporean context. By exploring sophisticated algorithmic strategies, traders can gain a deeper understanding of leveraging data-driven approaches for potentially more effective and efficient trading in this dynamic market.
Statistical arbitrage: Exploiting market anomalies
Statistical arbitrage is a quantitative strategy identifying temporary price discrepancies between related assets. This is achieved by analysing historical relationships between the prices of assets and executing trades when deviations occur. In Singapore, traders often apply statistical arbitrage to pairs of stocks or other financial instruments that exhibit high historical correlation.
For instance, if two stocks historically move in tandem but temporarily diverge in price, a statistical arbitrageur would buy the underperforming stock and short the outperforming one, anticipating reversing their historical relationship. This strategy requires advanced statistical modelling, real-time data feeds, and rapid execution capabilities to capitalise on fleeting opportunities.
Machine learning and predictive modelling: Forecasting market trends
Machine learning algorithms have gained prominence in quantitative trading for their ability to analyse vast amounts of data and extract meaningful patterns. In Singapore, traders utilise machine learning to develop predictive models that forecast market trends, price movements, and volatility. These models can process various factors, such as technical indicators, economic data, and news sentiment.
For example, a machine learning algorithm might analyse historical price patterns, trading volumes, and macroeconomic indicators to make short-term forecasts. Traders must backtest and validate their models to ensure their effectiveness in real-world trading scenarios. Machine learning-based strategies require continuous refinement and adaptation to evolving market conditions.
High-Frequency Trading (HFT): Capitalising on milliseconds
High-frequency trading involves executing many orders within fractions of a second to capitalise on minuscule price discrepancies. In Singapore, where cutting-edge technology infrastructure is prevalent, high-frequency trading firms compete to achieve the fastest execution times. This strategy often requires co-location with exchanges, low-latency trading platforms, and advanced algorithms.
Traders in this space may focus on market-making, providing liquidity by placing orders on both sides. By taking advantage of the bid-ask spread, high-frequency traders aim to generate small but consistent returns. However, it’s important to note that high-frequency trading is highly competitive and requires a deep understanding of market microstructure.
Quantitative portfolio management: Optimising risk-return profiles
Quantitative portfolio management involves applying mathematical models to optimise capital allocation across various assets. In Singapore, traders use techniques like mean-variance optimization and the Capital Market Line to construct portfolios that offer the highest expected return for a given level of risk.
For instance, a quantitative portfolio manager might use historical return data and volatility measures to determine the ideal weightings for different assets. Dynamic portfolio rebalancing based on changing market conditions is a crucial aspect of this strategy when traders buy stocks. By systematically adjusting portfolio allocations, traders aim to adapt to evolving market dynamics and optimise their risk-return profiles.
Sentiment analysis and news trading: Leveraging information flow
Sentiment analysis uses natural language processing and machine learning to assess public sentiment from news articles, social media, and other sources. In Singapore, traders leverage sentiment analysis to gauge market sentiment and make trading decisions based on the collective mood of market participants.
For example, if a positive news sentiment is detected regarding a particular stock, a trader might initiate a long position in anticipation of a price increase. However, it’s important to note that news-based trading carries inherent risks, as sentiment can change rapidly, and unexpected events may occur. Traders employing this strategy must have robust risk management practices in place.
Algorithmic risk management: Mitigating market volatility
In the realm of quantitative trading, managing risk is of paramount importance. Traders employ sophisticated algorithms to monitor market conditions in real-time and execute risk management strategies when necessary. These algorithms can automatically trigger stop-loss orders, adjust position sizes, or temporarily halt trading in response to adverse market movements.
For instance, in the event of sudden market volatility, a risk management algorithm might swiftly reduce exposure to higher-risk assets or move into safer, more defensive positions. Advanced risk models can help traders identify and quantify potential risks within their portfolios, allowing for proactive adjustments to maintain a desired risk profile. By incorporating robust risk management algorithms, traders in Singapore can navigate the market with greater confidence and resilience.
Final thoughts
Quantitative trading in Singapore represents a dynamic and evolving field characterised by the application of sophisticated algorithms and advanced data analysis techniques. Strategies like statistical arbitrage, machine learning, high-frequency trading, quantitative portfolio management, and sentiment analysis play pivotal roles in shaping the landscape of algorithmic trading in Singapore.
As traders venture into this realm, it’s essential to approach quantitative trading with a solid understanding of the strategies, rigorous backtesting, and a commitment to continuous learning and adaptation. By integrating these quantitative techniques, traders can confidently navigate the complexities of the Singaporean financial markets and potentially enhance their trading outcomes.